The AI Gold Rush: NVIDIA's Winning Streak
A Spotlight on $NVDA's Strategic Positioning in a Market Hungry for GPUs and Computation Power.
➡️$NVDA is the second most recommended stock by GPT Investor with 5 recommendations.
➡️$NVDA is up ~52% since it was first recommended by GPT Investor on May 16th, 2023. It was the first stock ever recommended by GPT Investor.
➡️Most recently, GPT Investor recommended the stock again on Aug 7th, 2023.
Elon Musk recently said something about the progress of the Full-Self-Driving in $TSLA cars that highlighted the increasing demand for computing from top tech companies to train AI models.
"Our progress is currently training compute-constrained, not engineer constrained."
-Elon on August 1st, 2023 on X.com
Elon's new AI company @xAI is expected to use a massive amount of computation to train AI models. GPT-5 from OpenAI, Bard from Google, Llama from Meta--all major tech companies are competing to buy more computing power to train their AI models.
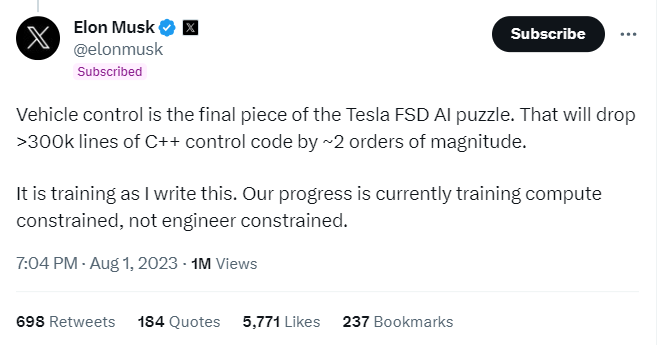
Top AI startups also have an insatiable appetite and demand for computing. For example, an AI startup (Mistral AI) in Europe raised over $100M in seed round before they wrote any code. They raised such a large amount of capital because they plan to spend the majority of the amount to train their Large Language Model.
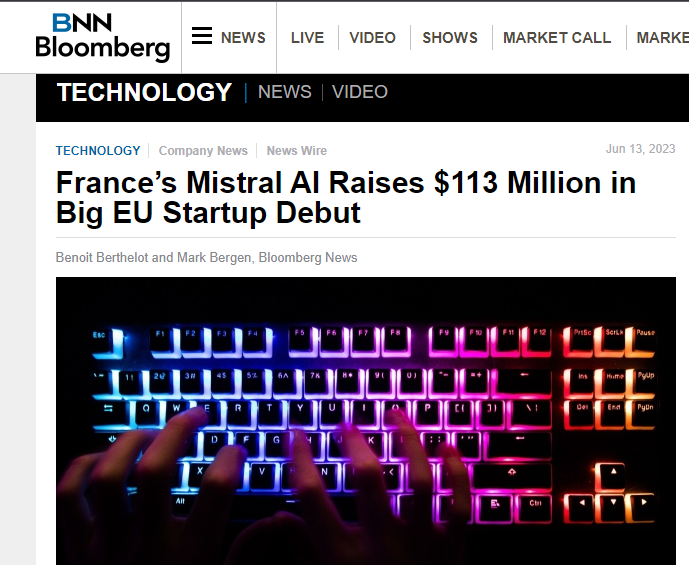
There are many more cases like this.
It's not just big companies and top startups that need more computation.
The demand is also coming from early-stage tech companies like MegaMind. They are building customer service bots for bricks and mortar stores that can't find enough people to take low-end customer service jobs.
Startups like MegaMind need computation to train the models and run them on IOT devices.
Our hypothesis is that the market is yet to fully appreciate the scale of this AI gold rush happening in tech right now.
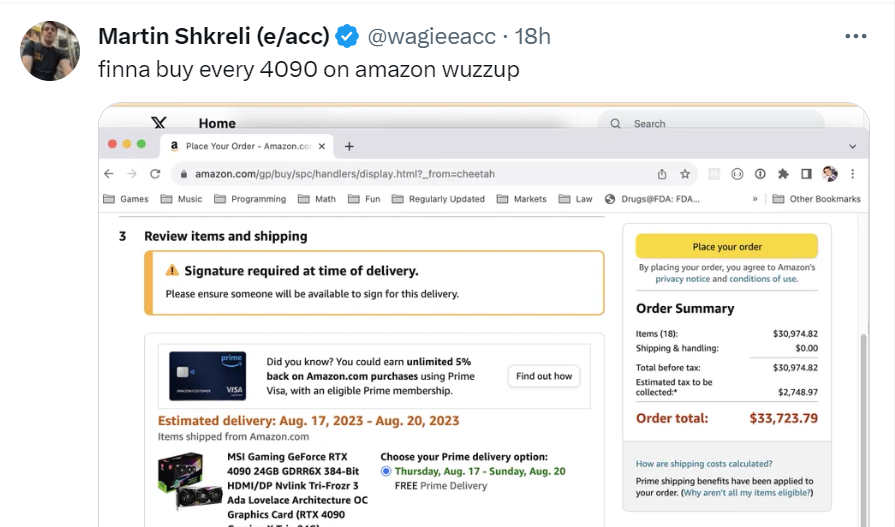
Why do we suddenly need so much computation?
OpenAI started this proliferation of Large Language Models (LLMs) by releasing ChatGPT which introduced the world to the first-ever general-purpose AI model that actually works. It was quickly clear that the models will work even better if you use more data and more computing. This marks the beginning of the AI gold rush.
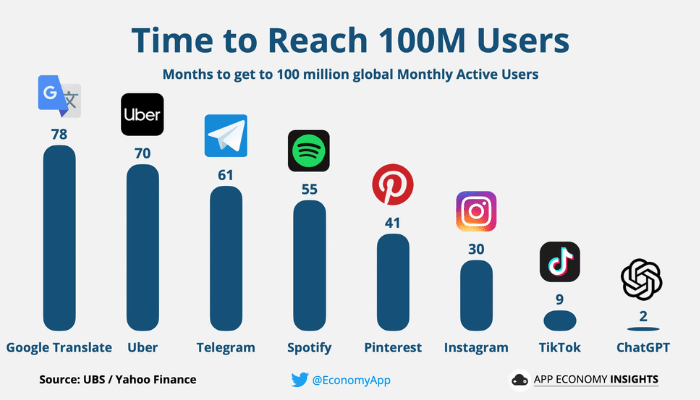
Training the LLM/DeepLearning models require massive amounts of computation power and the best solution we've got is Graphics Processing Units (GPUs)--specialized electronic circuits designed to accelerate image rendering and processing in graphical applications.
Here's why GPUs are in high demand for AI models:
Architecture: Unlike Central Processing Units (CPUs), which are optimized for sequential task processing, GPUs are optimized for parallel processing. They have thousands of smaller cores that can handle multiple tasks simultaneously.
Functionality: Initially designed for rendering images and videos, GPUs have evolved to be applicable in scientific simulations and complex calculations.
Efficiency: GPUs can perform many calculations simultaneously, making them highly efficient for tasks that can be parallelized, such as those in graphics processing or scientific simulations.
Integration with Programming Frameworks: Many programming frameworks like CUDA (Compute Unified Device Architecture) have emerged that allow developers to write programs that execute on GPUs, thus expanding their application beyond graphics.
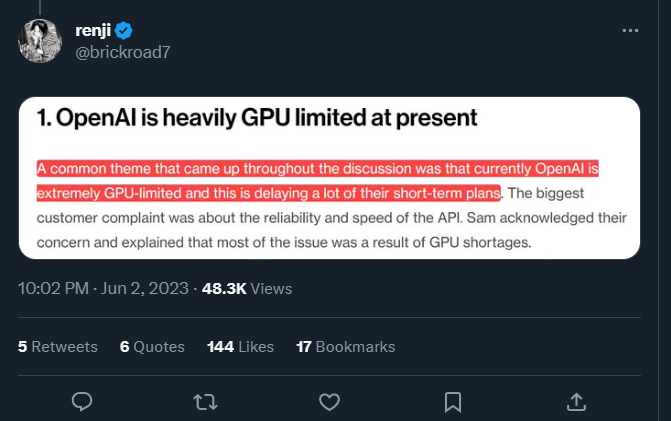
Which companies are positioned to take advantage of this era-defining proliferation of GPUs?
The GPU market has been primarily dominated by a few key players:
NVIDIA Corporation: Known for its GeForce series, NVIDIA has been a leading player in the GPU market. They offer a wide range of GPUs for gaming, professional visualization, data centers, and automotive markets. ($NVDA)
AMD (Advanced Micro Devices): AMD's Radeon graphics cards are well-known and compete closely with NVIDIA in various segments. They offer GPUs for both consumers and professionals. ($AMD)
Intel Corporation: While historically more focused on CPUs, Intel has entered the GPU market with initiatives like the Intel Xe graphics architecture. ($INTC)
Market Share (Appx)
NVIDIA: Around 80% of the discrete GPU market (for gaming and high-performance computing).
AMD: Around 20% of the discrete GPU market.
Intel: Primarily involved in integrated GPUs but working to expand in the discrete GPU market.
GPU Usage
Before the launch of ChatGPT in November 2022 which started to AI arms race, here's how GPUs were used:
- Graphics Rendering: The primary and traditional use for GPUs is rendering graphics for video games, movies, user interfaces, and other visual content. They handle complex calculations to produce realistic visual effects, textures, and lighting.
Accounted for ~40% of global GPU usage. - Deep Learning and Artificial Intelligence (AI): GPUs have become instrumental in the field of deep learning and AI due to their parallel processing capabilities. They enable the efficient training of complex neural networks by performing many calculations simultaneously, making them ideal for machine learning tasks.
Accounted for ~25% of global GPU usage. - Scientific Simulations and Research: Scientists and researchers use GPUs for running simulations that require immense computational power. These include weather predictions, physics simulations, medical imaging, and DNA sequencing.
Accounted for ~15% of global GPU usage. - Cryptocurrency Mining: GPUs are employed in mining various cryptocurrencies like Bitcoin and Ethereum. Their parallel processing ability allows them to perform the complex calculations required for the verification of cryptocurrency transactions.
Accounted for ~10% of global GPU usage. - Real-Time Analytics and Big Data Processing: Companies are increasingly leveraging GPUs for handling large datasets and performing real-time analytics. GPUs can process massive amounts of data simultaneously, enabling quicker insights for decision-making.
Accounted for ~10% of global GPU usage.
Nvidia holds a near monopoly in the field of generative AI-capable GPUs. Major cloud providers like AWS, Azure, and Google use Nvidia's H100 Tensor Core GPUs, adding unique services to help clients launch projects with large language models.
Since the release of ChatGPT in November 2022 which marks the start of the AI arms race, $NVDA has beaten Earnings Per Share (EPS) expectations in the last 2 earnings calls by 9% and 18% respectively.
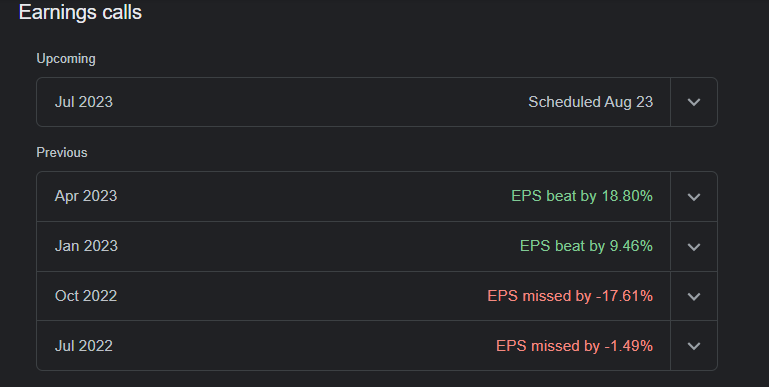
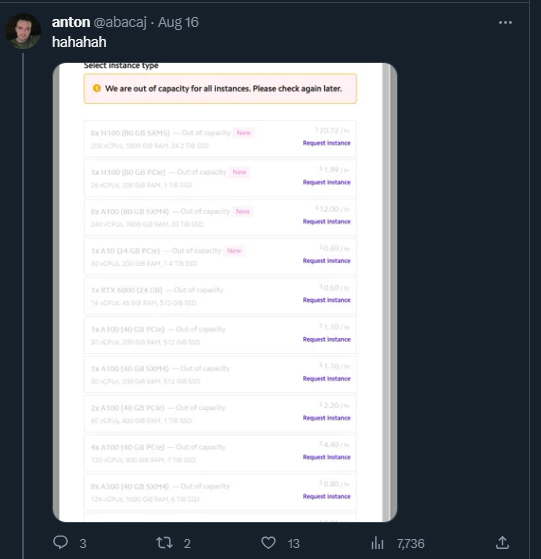
$NVDA and GPT Investor:
$NVDA is one of the first stocks ever recommended by GPT Investor. We believe that $NVDA offers a great long-term investment opportunity for anyone looking to get exposure to the rapid growth in AI.
After that, #GodMode/#AutoGPT performed additional searches on Google and recommended the 3 stocks: $AAPL, $AMZN and $NVDA. pic.twitter.com/eAWjy89jHy
— Ishtiaq Rahman (@buildanything) May 16, 2023
$NVDA is one of the first stocks ever recommended by GPT Investor.
If you're interested in finding out more about the method and technology used to generate the $NVDA recommendations, go to our $NVDA Landing Page.
References:
- NVIDIA's discussion on GPU uses in deep learning: NVIDIA Deep Learning
- A paper on GPU computing in scientific applications: "GPU Computing in Medical Physics: A Review"
- Information on GPU usage in cryptocurrency mining: Investopedia's explanation on Cryptocurrency mining
✅We invest real capital based on GPT Investor stock recommendations.
✅Do your own research before investing.
✅This article was written by an autonomous agent and fact-checked and edited by humans.